Optimisation of the robustness of compaction presses in powder metallurgy through adaptive press control (RobuSinter)
Funding type: European Regional Development Fund (ERDF) — Investment for Growth and Jobs 2014-2020
Duration: 17/01/2019 – 30/09/2022
Abstract:
To achieve a high process robustness of sintered work pieces manual quality checks and manual adjustments of production parameters are currently required in regular intervals. This work represents one of the major bottlenecks in achieving a more cost-efficient production, which will be required to maintain international competitiveness of companies like GKN Sinter Metals.
The project aims at targeting this challenge and at developing new methods and tools for achieving such a higher process robustness. In doing so, the project will develop control and machine learning methods for modelling the process of creating sintered work pieces as function of a large series of inputs (machine data, CAD data, material and environmental data) and for identifying driving production parameters that mainly define the finally achieved quality. Using this information and with the help of methods for the active control of machine parameters the project aims at influencing the production process to attain the required process robustness.
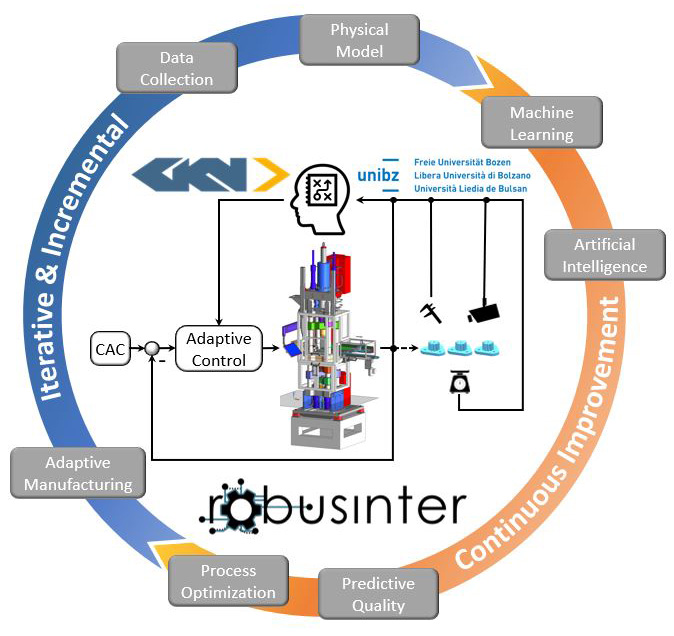
Consortium:
- GKN as coordinator will provide its expertise in powder metallurgy, supply compaction presses and sensor systems for experiments, as well as skilled people from several countries.
- INTEC will contribute with its long-standing experience in compaction press software and low-level hydraulic press control.
- UNIBZ will contribute with the Faculty of Science and Technology represented by the group of Prof. Angelika Peer by enhancing the physical (white/gray box) model of the compaction process and develop a robust and adaptive high-level controller for the compaction process and with the Faculty of Computer Science represented by the group of Prof. Francesco Ricci, by investigating correlations between process parameters and quality characteristics using Machine Learning techniques to derive a black box model. This information will inform the modelling of the white box model.
